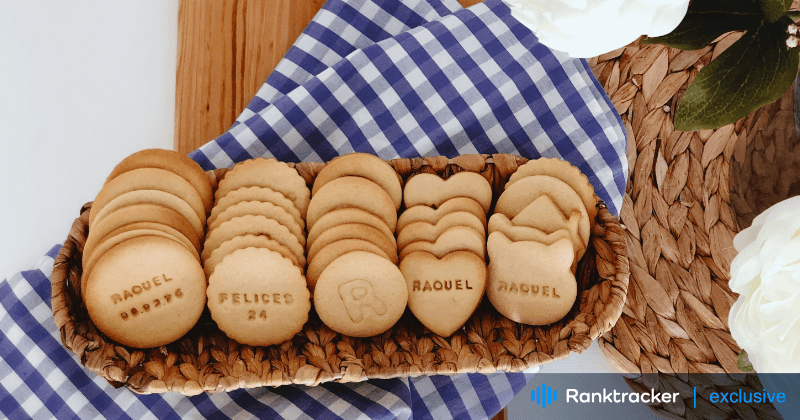
Intro
AI is reshaping the future of marketing. Today businesses rely on historical and real-time data to provide an incredible user experience and hyper-personalized product recommendations with AI.
Netflix is one of the notable brands pioneering hyper-personalized recommendations based on real-time data.
In this article, we will explain how AI provides a great customer experience, and why personalized product recommendations are crucial for improving the life value of a customer.
But, before that here is a list of interesting statistics that you should know,
Hyper-Personalized Product Recommendations Data Statistics
- 62% of customers expect brands to display personalized product recommendations to sustain brand loyalty.
- 49% of customers claim to become repeat buyers if businesses choose to offer hyper-personalized products.
AI-Powered Data Analysis
Data is the backbone of AI. The amount of data generated on a daily basis is, 328.77 million terabytes of data. This gives marketers incredible opportunities to study the target audience and their preferences.
This infographic from ZDNET displays everything that we should know as marketers. It reveals the life cycle of data from collection to its decision-making.
The All-in-One Platform for Effective SEO
Behind every successful business is a strong SEO campaign. But with countless optimization tools and techniques out there to choose from, it can be hard to know where to start. Well, fear no more, cause I've got just the thing to help. Presenting the Ranktracker all-in-one platform for effective SEO
We have finally opened registration to Ranktracker absolutely free!
Create a free accountOr Sign in using your credentials
Data Collection and Processing
Data is collected through diverse sources. Some of the significant data sources marketers use include;
- Cloud services include CRM, services, cases, digital footprints, tracking, eCommerce, social media insights, external insights, etc.
- Mobile, web, and devices that can provide data about app interaction, location, click patterns, and contextual data
- Enterprise systems that consist of a system of records, end-to-end journey data
- Virtual Systems including AR/VR technologies, metaverse, etc.
- The large sets of data collected are analyzed using advanced technologies, artificial intelligence, machine learning, and deep learning to give customers hyper-personalized recommendations.
Advanced Analytics for Customer Insights
To get advanced analytics for customer insights marketers need to gather data on the following parameters;
- Demographics and psychographics - It gives a wholesome approach to the ideal customer including its location, gender, age, income, job, interests, personal preferences, and lifestyle and values.
- Behavioral data - It includes the behavior of online shoppers including product purchases, abandoned cards, browsing history, and clicks.
- Transaction history - Purchase history includes the number of purchases, their frequency, and the types of items purchased.
- Interaction data - It includes all the engagement rates both on social media and the website, including bounce rates, email open rates, shares, comments, likes, followers, etc.
- Sentimental analysis - This is the measure of how satisfied your customers are with the product. It includes parameters like customer feedback and reviews on your product pages.
Real-time Data Utilization
AI enables businesses to ensure real-time processing and data analysis. As a result, they respond in real-time to ensure hyper-personalized product recommendations.
The key is to display the right product to the customer in real-time. This means if a customer is looking for a bike helmet on Amazon, it displays the best product along with some incentive to the ideal customer making the purchase irresistible, and the buying journey seamless.
Just look at this personalized offer with a “free delivery” option. This improves customer engagement and loyalty and entices the visitor to take action.
Tailoring Recommendations through Machine Learning
Predictive Modeling for Customer Preferences
Let's put this simply.
Machine learning algorithms use large sets of data to help you understand future customer preferences to drive hyper-personalization product recommendations. It uses a mathematical model to predict future customer trends, preferences, and customer behavior based on previous and current data.
ML can predict and estimate the engagement rates and quality of the leads on the specific product page. It can also tell you the actual outcomes. For example, machine learning can help you predict how many product returns will be in the future (in case there are any product returns in the past). This allows marketers to focus and promote the products that sell the best.
Contextual Analysis for Relevant Suggestions
Contextual analysis brings up products based on specific context. It takes the relevant data points to give appropriate suggestions.
The All-in-One Platform for Effective SEO
Behind every successful business is a strong SEO campaign. But with countless optimization tools and techniques out there to choose from, it can be hard to know where to start. Well, fear no more, cause I've got just the thing to help. Presenting the Ranktracker all-in-one platform for effective SEO
We have finally opened registration to Ranktracker absolutely free!
Create a free accountOr Sign in using your credentials
Contextual analysis drives insights based on the specific product feature the audience is discussing or talking about. Machine learning algorithms use advanced technology to turn every query into a single data point, analyze the data, and showcase relevant suggestions.
For example, eBay uses ML to segment customer queries based on price, including discounts, promotions, and special offers. And, displays products accordingly.
Natural Language Processing (NLP) in Personalization
NLP in personalization extracts insights from customer communication expressed through text, and visuals to display product recommendations.
Sentiment Analysis for Enhanced Recommendations
As its name suggests sentiment analysis is the measure of how satisfied your customers are with the product. It’s a textual analysis of emotions, attitudes, and feelings, expressed through text/words based on customer feedback and reviews on your product pages.
Sentiment analysis uses NLP which segments different data points based on text. The text is classified into negative, neutral, or positive sentences. Brands leverage user-generated content and analyze it through the following methods to provide hyper-personalized recommendations;
- Deep learning techniques
- Rule-based methods
- Machine learning techniques
- Sentiment strength
- Detection methods
- Swarm intelligence-based methods
- Sentiment lexicon expansion methods
- Bayesian methods
- Pattern-based methods
Predictive Analysis
Basically, NLP focuses on “next word prediction” that mimics human speech. The model is trained to analyze the sequence of sentences from the input and predict the text or words. As a result, it provides answers to the user queries in the most accurate way improving the probability of conversion rates.
A great application of NLP for predictive analysis is chatbots and virtual assistants. They use natural language generation (NLG) to create conversational responses to customer queries.
Chatbots and Virtual Assistants for Real-time Engagement
Both virtual assistants and chatbots use NLP and AI to convert text, and voice queries into structured data.
- Chatbots answer questions in real time.
- Virtual assistants perform administrative tasks.
They use advanced technology to understand queries or requests from the user and provide the answers in real-time. Chatbots and virtual assistants provide a personalized experience on various platforms by responding to emails, scheduling meetings, managing customer requests, answering queries, booking reservations, etc.
68% of customers love chatbots because of their efficiency and real-time engagement. They strengthen brand credibility, and loyalty with uninterrupted customer engagement, increased lead generation, and personalized recommendations.
Both Siri and Alexa are prime examples of virtual customer assistants that provide seamless customer experience.
Image Recognition and Visual Preferences
Visual Data Interpretation
Image recognition uses machine learning and deep learning to detect and identify an object and its features in a digital image. It recognizes a data set of images, recognizes patterns, and identifies different objects.
The image recognition feature of deep learning is impressive. It can identify any image and its context. For example, deep learning can tell you whether your furry friend is sleeping or just sitting on your couch.
The technology uses large sets of visual images and analyzes them to improve the efficiency and accuracy of image recognition significantly. The more data, the better!
Image-based Recommendation Algorithms
Based on the browsing history of the visual content on platforms like Pinterest, AI recommends the right kind of content to the audience. AI suggests personalized products by recognizing the kinds of products customers interact with giving a personalized experience like never before.
Google Lens
Google's Lens has transformed visual content search using image recognition technology. It uses the input analysis using ML and DL and provides personalized search results and information.
You can drag or upload an image to the Google Lens, and click on the “search” option to see all the relevant recommendations.
Enhancing Recommendations with Visual Input
Another great example of enhancing recommendations with visual input is ASOS, the famous fashion brand!
ASOS
ASOS uses AI to enhance product recommendations with visual input. The “Style Match” feature of the famous fashion retail allows the users to upload an image and display the right products accelerating the buying journey.
This feature is available on the iOS and Android ASOS App for now.
Reinforcement Learning for Adaptive Recommendations
Implementing AI for hyper-personalization product recommendations provides an incredible avenue for continuous learning from user feedback.
Based on the adaptive recommendations to the evolving preferences, businesses can provide the right kind of products to the right audience.
However, it’s crucial to balance exploration and exploitation when hyper-personalizing product recommendations.
Overcoming Challenges and Ensuring Privacy
Data Collection & Data Analysis
Data is precious, it provides a lot of opportunities for marketers. However, the real challenge is data collection and data analysis. Marketers must rely on advanced systems like cloud services, mobile, and web devices, enterprise systems, and virtual systems to capture data points, and then analyze them.
The All-in-One Platform for Effective SEO
Behind every successful business is a strong SEO campaign. But with countless optimization tools and techniques out there to choose from, it can be hard to know where to start. Well, fear no more, cause I've got just the thing to help. Presenting the Ranktracker all-in-one platform for effective SEO
We have finally opened registration to Ranktracker absolutely free!
Create a free accountOr Sign in using your credentials
Secondly, data is collected from various sources, therefore it turns out to be very fragmented. Analyzing this data using one method gives biased results. Mere human capacity is not enough to analyze data therefore businesses need to use advanced technologies like AI, ML, and Deep Learning.
Dealing with Data Quality and Bias Issues
Quality data is the key to AI’s efficiency. If the data in consideration is poorly labeled, the results could be inaccurate. Marketers can overcome this by tagging the data correctly, be it text, images, or other visuals to avoid biased results.
Addressing Scalability and Infrastructure Requirements
Scaling your businesses using AI is daunting, it requires input from both the human resources that you use, and the infrastructure including systems, and software.
Addressing the Privacy Concerns
There is a significant risk of privacy breaches when tackling data at a large scale. To sustain customer loyalty and trust make sure you communicate the transparency of data ahead of time. Businesses need to comply with the regulations including CCPA, GDPR, etc.
Future Directions of Hyper-Personalization
Integration of AI with IoT Devices
AI is not a mere revolution, it’s an entire evolution. This cutting-edge technology is going even further in providing a laser-focused personalized experience with the integration of AI with IoT Devices.
Personalized Health and Wellness Recommendations
Hyper-personalization is becoming popular across all industries, particularly in health and wellness.
These applications use granular level data to offer personalized recommendations like workouts, diet, and nutritional plans based on various parameters like,
- Hormonal profiles
- The emotional state of the individuals
- Sentimental analysis
Predictive Personalization in Emerging Industries
With the potential that AI offers to businesses, it will help them get rid of the “one size fits all” approach across emerging industries.
With its advanced technology, AI has disrupted industries including health care, fitness, sports, beauty and wellness, etc. In the future, AI will enable brands to provide recommendations based on real-time data, and it can even provide based on facial recognition to give accurate recommendations.
Conclusion
AI brings a gold mine of opportunities enabling businesses to laser-focused personalized product recommendations to increase ROI and decrease customer acquisition costs.
Businesses leveraging and adapting to AI trends and technologies succeed at providing a winning customer experience. While AI brings a lot of challenges associated with data, businesses need to empower themselves with the right resources and systems to scale seamlessly.